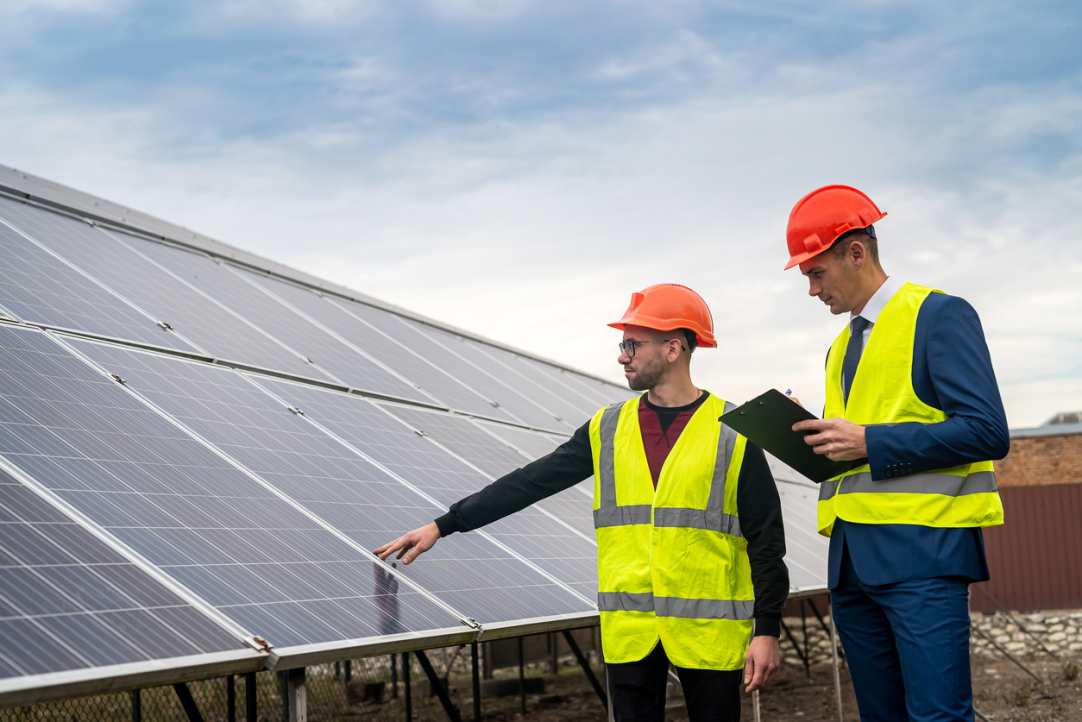
Machine Learning Helps Improve Perovskite Solar Cells
A team of researchers from HSE MIEM, LPI RAS, and the University of Southern California have applied machine learning to the analysis of internal defects in perovskite solar cells and proposed ways to improve their energy efficiency. The findings of the study performed on the Cs2AgBiBr6 double perovskite can be used to develop more efficient and durable perovskite-based materials. The paper has been published in the Journal of Physical Chemistry Letters.
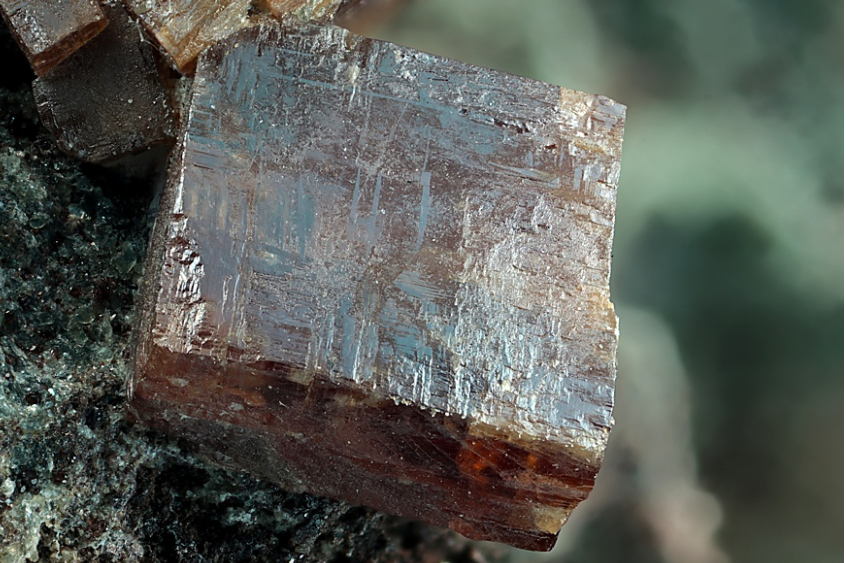
New Data Gained on Double Perovskite Oxides
The Journal of Alloys and Compounds has published an article coauthored by the Institute of Solid State Chemistry and Mechanochemistry (the Ural Branch of the Russian Academy of Sciences), the Donostia International Physics Centre, and the HSE Tikhonov Moscow Institute of Electronics and Mathematics on the characteristics of cubic double perovskite oxides. To date, experimental measurements of the minerals’ characteristics have not corresponded to the results of theoretical modelling. The work marks the first time that researchers have set themselves the task of explaining this disparity. The data obtained will allow researchers to improve low-temperature fuel cell technologies—one of the main alternatives to current sources of electricity.